Erklärbare KI für automatisierte Produktionssysteme
Laufzeit: 01.01.2020 - 31.12.2021
Partner: Bundesministerium für Bildung und Forschung (Fördergeber), Old World Computing GmbH, iTAC Software AG, Hella GmbH & Co. KGaA, Universität Stuttgart, Fraunhofer ISST
Förderung durch: Bundesministerium für Bildung und Forschung Deutsches Zentrum für Luft- und Raumfahrt
Kurzfassung
Automated production systems (aPS) organize operating resources in complex processes. These resources handle intermediate products and check their quality. Depending on the test result, an intermediate product is passed on in the process, scrapped or rejected for manual testing and reworking. Although the individual steps are monitored, there are such complex interdependencies between material, processing steps, equipment, equipment maintenance and test results that even if the intermediate...
Automated production systems (aPS) organize operating resources in complex processes. These resources handle intermediate products and check their quality. Depending on the test result, an intermediate product is passed on in the process, scrapped or rejected for manual testing and reworking. Although the individual steps are monitored, there are such complex interdependencies between material, processing steps, equipment, equipment maintenance and test results that even if the intermediate products are supposedly processed correctly, an end product may lack the required quality, resulting in expensive reworking or even rejects. The aim of the project is not only to detect complex dependencies between errors and processing at an early stage by means of machine learning, but also to explain these dependencies by means of AI in such a way that the operator can optimize his aPS efficiently and effectively. For this purpose, XAPS combines existing descriptions of digital factories and digital twins from the Manufacturing Execution System (MES) with formal AI methods, machine learning (ML) and domain modeling. This provides the basis for a holistic solution portfolio for controlling and monitoring aPS, which addresses a market for solution providers that is worth €15 billion globally for electronics manufacturing and as much as €454.5 billion in the automotive sector.
Within this framework, the RGSE research group is researching methods and tools to specify and analyze relationships between digital twins of plants, machines, parts and products, process descriptions and process instances.
» weiterlesen» einklappen
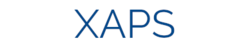