Semi-Automatic Particle Tracking for and Visualization of Particle Detector Data
Bender, Jan; Botsch, Mario; Keim, Daniel A. (Hrsg). Proceedings Vision, Modeling, and Visualization. Genf: The Eurographics Association 2022 8 S.
Erscheinungsjahr: 2022
ISBN/ISSN: 978-3-03868-189-2
Publikationstyp: Diverses (Konferenzbeitrag)
Sprache: Englisch
Doi/URN: 10.2312/vmv.20221210
Inhaltszusammenfassung
In high energy physics, tracking particles in point-based particle detector data is important to reconstruct the particles’ trajectories. As the numbers of particles can be in the hundreds or over a thousand, automatic algorithmic tracking is usually preferred over manual tracking. However, in some cases, manual tracking is needed as a baseline to assess the quality of the algorithmic tracking. Tracking particle locations manually is time-consuming and challenging when dealing with thousan...In high energy physics, tracking particles in point-based particle detector data is important to reconstruct the particles’ trajectories. As the numbers of particles can be in the hundreds or over a thousand, automatic algorithmic tracking is usually preferred over manual tracking. However, in some cases, manual tracking is needed as a baseline to assess the quality of the algorithmic tracking. Tracking particle locations manually is time-consuming and challenging when dealing with thousands of particles in the same data frame. In this paper, we describe Semi-Automatic Particle Tracking (SAPT), a collection of methods that aid manual particle tracking and visualization. These methods aim to make related particle hits easier to recognize by stretching the data and hiding likely irrelevant hits based on an angle criterion. They also help with finding the most likely track among a set of intuitively selected detector hits. These methods, together with a prediction of the most probable continuation of a track that can simply be accepted by the human user, accelerate the manual tracking process tremendously. We demonstrate the usefulness and efficiency of our methods by applying them to simulation data of a detector for proton computed tomography (pCT).» weiterlesen» einklappen
Autoren
Klassifikation
DFG Fachgebiet:
Informatik
DDC Sachgruppe:
Informatik
Verbundene Forschungsprojekte

Verknüpfte Personen
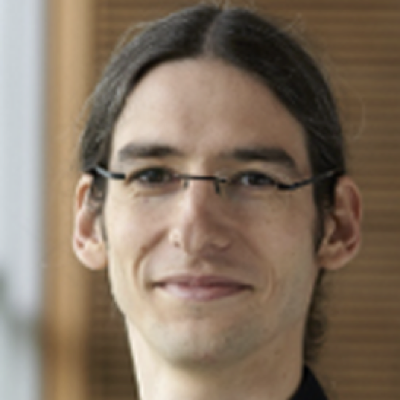
- Alexander Wiebel
- Professor
(Informatik)

- Ralf Keidel
- Hauptforscher SIVERT
(Zentrum für Technologie und Transfer | ZTT)