Investigating particle track topology for range telescopes in particle radiography using convolutional neural networks
Acta Oncologica. Bd. 60. H. 11. Taylor & Francis 2021 S. 1413 - 1418
Erscheinungsjahr: 2021
Publikationstyp: Zeitschriftenaufsatz
Sprache: Englisch
Doi/URN: 10.1080/0284186x.2021.1949037
Geprüft | Bibliothek |
Inhaltszusammenfassung
Background Proton computed tomography (pCT) and radiography (pRad) are proposed modalities for improved treatment plan accuracy and in situ treatment validation in proton therapy. The pCT system of the Bergen pCT collaboration is able to handle very high particle intensities by means of track reconstruction. However, incorrectly reconstructed and secondary tracks degrade the image quality. We have investigated whether a convolutional neural network (CNN)-based filter is able to improve the...Background Proton computed tomography (pCT) and radiography (pRad) are proposed modalities for improved treatment plan accuracy and in situ treatment validation in proton therapy. The pCT system of the Bergen pCT collaboration is able to handle very high particle intensities by means of track reconstruction. However, incorrectly reconstructed and secondary tracks degrade the image quality. We have investigated whether a convolutional neural network (CNN)-based filter is able to improve the image quality. Material and methods The CNN was trained by simulation and reconstruction of tens of millions of proton and helium tracks. The CNN filter was then compared to simple energy loss threshold methods using the Area Under the Receiver Operating Characteristics curve (AUROC), and by comparing the image quality and Water Equivalent Path Length (WEPL) error of proton and helium radiographs filtered with the same methods. Results The CNN method led to a considerable improvement of the AUROC, from 74.3% to 97.5% with protons and from 94.2% to 99.5% with helium. The CNN filtering reduced the WEPL error in the helium radiograph from 1.03 mm to 0.93 mm while no improvement was seen in the CNN filtered pRads. Conclusion The CNN improved the filtering of proton and helium tracks. Only in the helium radiograph did this lead to improved image quality.» weiterlesen» einklappen
Autoren
Klassifikation
DFG Fachgebiet:
Informatik
DDC Sachgruppe:
Informatik
Verbundene Forschungsprojekte

Verknüpfte Personen

- Steffen Wendzel
- ehemaliger Wissenschaftlicher Leiter
(Zentrum für Technologie und Transfer | ZTT)

- Ralf Keidel
- Hauptforscher SIVERT
(Zentrum für Technologie und Transfer | ZTT)
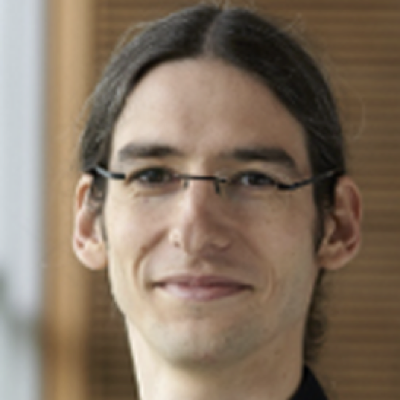
- Alexander Wiebel
- Professor
(Informatik)